A major step forward in creating intelligent robots-ones that learn to perform tasks without having to be programmed-will be unveiled this weekend at the International Conference on Robotics and Automation in Seattle.
Those in attendance will witness BRETT, which stands for Berkeley Robot for the Elimination of Tedious Tasks, complete a series of tasks without having been pre-programmed.
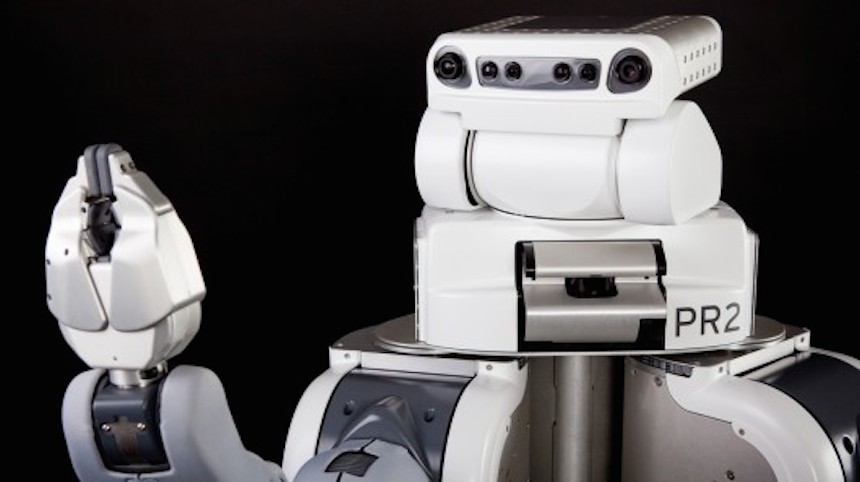
Traditional robot programming involves inputting details about the tasks including possible scenarios and outcomes. This voluminous method requires lots of storage, programming hours, and is limited by the imaginations of the programmers and the amount of controls that can be placed on the environment.
BRETT was programmed with an artificial intelligence concept known as "deep learning," which draws on the human brain's neural net as its model for processing information from the outside world. In the deep learning design, artificial "neurons" are layered to process sound or image data in sets of patterns or categories, which helps the robot "understand" the data it receives in real time.
Early advances in this type of data reading have been popularized by voice and sight recognition programs like Siri or Google Street View. BRETT moves far beyond passive processing of sound and images, however, and applies deep learning to motor tasks that engage the world around it.
"Moving about in an unstructured 3D environment is a whole different ballgame," said Chelsea Finn, a member of the University of California, Berkeley, team responsible for the project. "There are no labeled directions, no examples of how to solve the problem in advance. There are no examples of the correct solution like one would have in speech- and vision-recognition programs."
The researchers are working through the UC's Center for Information Technology Research in the Interest of Society (CITRIS), a multidisciplinary, multi-campus effort to corral advances in robotics and artificial intelligence-and keep them focused on improving the lives of human beings.
This project-part of a People and Robots Initiative-refined reinforcement learning to the degree that the robot completes tasks such as placing a clothes hanger on a rack, assembling a toy airplane, and screwing a cap on a water bottle, all without being pre-programmed to interact with its surroundings.
"The key is that when a robot is faced with something new, we won't have to reprogram it. The exact same software, which encodes how the robot can learn, was used to allow the robot to learn all the different tasks we gave it," said project co-leader Pieter Abbeel of UC Berkeley's Department of Electrical Engineering and Computer Sciences.
Placing a clothes hanger on a rack is a long way from sorting the laundry, of course, but BRETT represents the type of advancement necessary to place a robot in a real-life setting, where environments are dynamic, and expecting the robot to adapt successfully to its surroundings.
"Our initial results indicate that these kinds of deep learning techniques can have a transformative effect in terms of enabling robots to learn complex tasks entirely from scratch," said Abbeel. "In the next five to 10 years, we may see significant advances in robot learning capabilities through this line of work."
In the meantime, BRETT-which is based on a Willow Garage Personal Robot 2 (PR2)-uses a camera to assess the position of its own arms and hands. Based on movements that bring it closer to completing a task, BRETT's algorithm calculates values for some 92,000 parameters and provides real-time feedback through its neural net. In this way BRETT learn which movements are better suited for the task it's performing.
"For all our versatility, humans are not born with a repertoire of behaviors that can be deployed like a Swiss army knife, and we do not need to be programmed," explained team member Sergey Levine. "Instead, we learn new skills over the course of our life from experience and from other humans. This learning process is so deeply rooted in our nervous system, that we cannot even communicate to another person precisely how the resulting skill should be executed. We can at best hope to offer pointers and guidance as they learn it on their own."
Follow Will Hector on Twitter: @WriterWithHeart
(Sources: Science Daily; ICRA2015.org)